Navigating AI Adoption: The Build versus Buy Conundrum
Add bookmark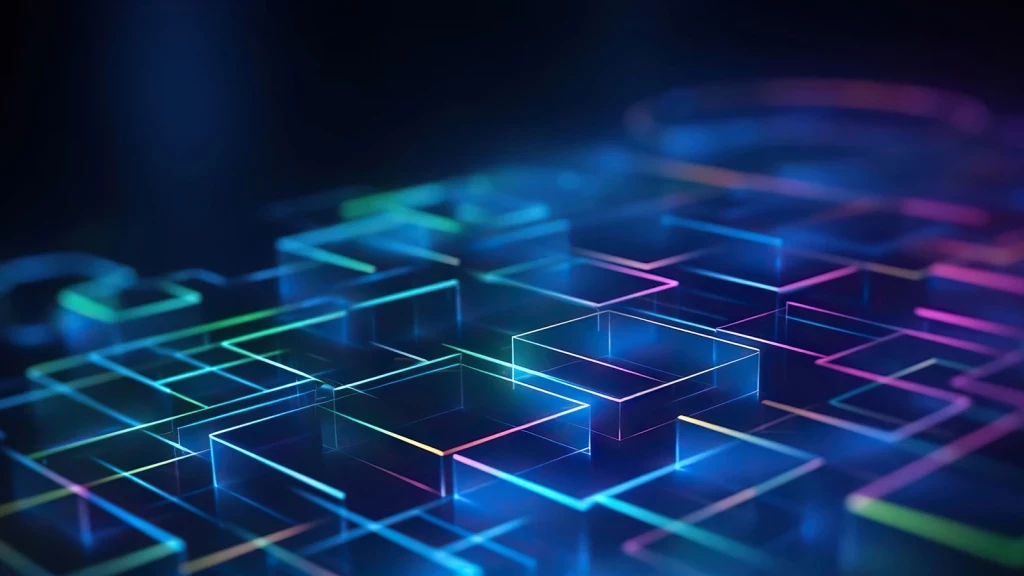
No longer a nice-to-have, AI is well and truly here. The market is moving at a frenzied pace.
A significant three in four CX leaders report that investing in CX-focused AI technology is high on the agenda of their C-suite right now, with more than one in five saying it is “very high”. As a result, there are CX decision-makers and project heads everywhere in the process of adopting AI-driven tools into their CX ecosystems. And with that comes a key dilemma: to look for an off-the-shelf solution or to construct something entirely in-house.
Here, we speak with two leaders who have gone down opposing paths. In the “build” corner we have Paul Cooper, the former Head of Technology Delivery, Operations and Cyber at takepayments, and in the “buy” corner we have Dan Allen, the Deputy Director of Landlord Support at the National Residential Landlords Association (NRLA). Together they look at the pros and cons of each approach and offer critical insights around AI scalability, maintaining AI independence, implementation best practices, talent management, and their biggest learnings.
Simon Hall: Dan, Paul – Welcome, great to have you. It’s well established now that investing in CX-focused AI is a competitive imperative for brands. And against that mandate, a variation of the age-old question around whether to build or to buy is very much top of mind for CX leaders when it comes to developing their strategies with – and approach to – this technology. That’s the theme we’ll be exploring here.
So, to set up our conversation, can I ask you both to unpack your respective journeys with AI so far. Dan, let’s kick off with you...
Dan Allen: Absolutely! Here at the NRLA we've been fostering a culture and a passion for AI adoption across our organisation for well over a year. Our main focus has been to engage employees across all departments, and then understand and identify the opportunities that exist to leverage the technology to improve our member experience.
We’ve taken a marginal gains rather than a big bang approach to adoption. We firmly believe that AI offers myriad use cases to enhance our operational processes, yet we’re very much coming at this from a human-in-the-loop perspective. And we’ve had varying degrees of success; we’ve had some false starts, and we’ve also had some wins. Over the last 12 months, we’ve actively sought insights from experts in the space and we’ve spoken to a series of vendors and software developers – all to understand where best to position ourselves within the realm of AI.
SH: And you Paul?
Paul Cooper: In recent years, there has been a notable surge in the enthusiasm surrounding AI models – tools like ChatGPT have garnered widespread attention. I embarked on a similar approach to Dan in that we rolled out closed-door experiments to discern and understand the specific issues these solutions could address.
There’s always a danger when deploying technology – especially nascent technology like this – without first clearly defining the problems they aim to solve. With the prevalence of ChatGPT and the like now, there is a real risk of grey IT popping up all over the place with these models so we’re trying to stay ahead of that through education – we're communicating the value that AI can deliver.
We’re primarily utilising it to improve internal productivity. Just by maintaining that sole line of sight for now, we can exercise greater control and mitigate any risks to the business – those errant conversations or unintended customer interactions that can spring up when these programmes are placed directly in front of customers. The technology isn’t ready for that yet.
SH: Regardless of whether organisations opt for developing a solution in-house or working with a third-party provider, the complexities involved in implementing AI, in either capacity, are many. What are the core factors that CX leaders should take into consideration when deciding between building or buying their AI technology?
DA: It’s important to be honest with yourself about your capabilities and what you can achieve in a particular space. One of the major benefits of buying is that providers often offer a one-to-many solution, which can be purchased at a more affordable rate without spending a lot of time on development or facing the possibility of multiple false starts. Mistakes can be costly – especially for member organisations like ours that are not-for-profit. We don’t want to waste our members’ money, and that may seem a risk-averse approach, but it brings significant advantages.
The biggest drawback, of course, is that you’re not designing for your company and your unique business model – you have less control over exactly what it does and elements around that. That’s the trade-off CX leaders need to make.
PC: The landscape of language and AI models has been evolving quickly through the likes of OpenAI and Anthropic, who are pioneering the development of highly complex and costly models. In that process, they’re creating ecosystems wherein their mechanics can be shaped and adapted by others. And that extension piece is where the gold is: It negates the need for comprehensive training and instead emphasises the importance of understanding the specific problem at hand and customising data sets to enable the models to be employed in a specific context.
There’s definitely value to that given much of the cost risk has already been absorbed by these companies. Granted they’re passing that cost on to users to some degree – it's not free to use some of these tools – but nonetheless, the ecosystem of models and smaller training sets present numerous opportunities.
Conversely, there are organisations like Facebook that are releasing entirely open-source models. I certainly see the mileage in that as well, but then it shifts the associated risks even further. The crucial question that arises in all of this is around the level of trust businesses can place in the training data of these models – that demands careful consideration.
SH: A proposed key benefit of going down the open-source route is that it enables CX teams to retain control and adapt their AI training models and their methodologies to their specific business needs. Paul: Given that you have taken the path to build, how do you evaluate the long-term scalability and flexibility of this approach?
PC: The field is highly specialised, which has limited its scalability. Recruiting top-tier talent has proven to be a challenge, but it is getting easier.
These tools are progressively becoming more user-friendly, and as a result, we’re seeing an increase in the number of data scientists in the market. The entry barriers to working with AI are diminishing with each passing year. It remains a niche space, though, and sourcing the best data scientists continues to be a difficult task.
The long-term scalability and sustainability of this technology are on the rise as it continues to advance, thereby mitigating some of the associated risks. If you have the resources and the right skill sets within your team, it makes a lot of sense to invest in individuals who can custom-tailor solutions to tackle your unique challenges as opposed to buying off-the-shelf solutions.
SH: Paul, sticking with you: It’s nothing short of critical that brands incorporate a comprehensive risk assessment into their AI roadmaps so that they can protect and prepare themselves as best as possible. It’s important to have both guardrails in place and the capacity to scale. With that said, what risks have you identified in relying solely on open-source models?
PC: The insights. The outputs. These models have not yet reached a stage where I would feel entirely comfortable allowing them to directly interact with a customer – there still always needs to be a human-in-the-loop, which goes back to what Dan mentioned earlier.
When considering these risks, it’s imperative to maintain absolute clarity regarding your data. If you grant an AI algorithm access to your data, it’s crucial to meticulously define the labeling and access parameters. You don’t want a scenario whereby it’s given permission to see sensitive information, or data protected by regulation, so organisations need to build a very comprehensive data and labeling strategy behind the scenes.
And that responsibility extends beyond merely automating processes. It demands a concerted effort to educate employees around data handling practices. There should also be heightened concerns pertaining to data governance – if you don’t get that piece of the puzzle right, then the model may start engaging in activities you don’t want it to. That’s the primary area of concern right now.
SH: Dan, let’s turn over to you. The AI ecosystem is moving and evolving in such a way, at such a pace, that any AI partnership needs to transcend any conventional vendor/brand relationship. There needs to be an enhanced level of trust and a deep level of strategic and tactical collaboration as the space and the technology continuously mature into unknown territory. What key components were you looking for when you were on the hunt for a vendor?
DA: We’re looking for people who share our perception of exceptional service, a standard that should be universal but often isn’t. We’ve chosen to partner with smaller players because they are more attuned to our objectives and how we intend to leverage the technology. Our core focus revolves around several key questions: Will it enhance operations for our members? Will it streamline our business processes? Will it improve accessibility and interaction for all parties involved? Will it help support our colleagues and/or members?
Our emphasis is not solely on productivity or cost-saving measures, although these are both positive byproducts of implementing AI technology. And, of course, they are a partial consideration, and we monitor both. The key point, though, is the motivation of the vendor. Are they driven solely by the desire to create a business case that saves us money, or are they dedicated to enhancing the experience for our members? If our objectives for the technology align – a determination drawn from extensive discussions – then those are the companies we want partner with and procure from.
Ultimately, the decision hinges on whether their ethos harmonises closely with ours.
SH: And Dan, how do you strike a good balance between allying with your AI vendor and maintaining independence? What work are you doing to ensure that all the AI work you’re doing now – building the right architecture, building capabilities – is sustainable and viable in the long-term, three, five, 10 years from now?
DA: It's a really good question.
We’re currently in the midst of a range of activities and we’re looking to do more. Like Paul, maintaining the accuracy and reliability of our data is a top priority, but in a different way. We offer an advisory service as part of our membership – our knowledge and our experience and our insights are the products we’re selling. We’ve been on a significant journey over the last 12 months to document as much as we can in a clear format, in a central knowledge base, that can serve as a repository for AI access.
In essence, we’re not developing the AI itself, but rather the content that’s being fed into the technology. And this represents a huge challenge because we work in an industry that’s ever changing. For example, just recently, new legislation was announced that will completely change the game for the rental market in the U.K. Overnight, around 70% of our existing knowledge will be rendered obsolete. It’s going to take an incredible effort to redocument the insights. It’s our data and knowledge that are special to us – and it’s critical that we continue to develop both over the next three, five, 10 years.
Irrespective of our future partnerships or AI service providers, our objective will be for them to seamlessly plug into what makes us special and enhance that.
SH: To get AI implementation right, enterprises need to master various elements simultaneously, chief among them structuring unstructured data, developing frameworks to access quality data, and putting up walls to establish its safe implementation. Question for both of you now: What best practices should brands adhere to for each approach – open-source versus third-party – when it comes to data security?
PC: Regardless of your approach, the internal strategy for ensuring data security is likely to be the same. You need a strong handle over key data sets, their sources, and their intrinsic value – you need that central control. You can’t have fragmented pockets of information and expect an AI tool to work everything out for you.
Right now, we have a multitude of similar knowledge bases – and those knowledge bases have originated from humans. However, here itself there is a risk emerging. People in our organisation, across disparate teams, are using AI to create knowledge articles and craft policies. Consequently, then, our data sets are going to have AI generated knowledge in there as well. This prompts the question of how far we should proceed in this direction. If hands are completely off the wheel and control is relinquished to AI, then we’ll be left in a self-perpetuating cycle.
As leaders, we need to be thinking forward and staying cognizant of such potential risks. We need to ensure there are guardrails in there – checks and balances around what’s going into AI.
DA: I echo Paul’s sentiments around the necessity of establishing appropriate policies and procedures.
Effective governance over data should be universal regardless of whether you’re developing or procuring solutions. It can’t be understated how robust the foundations must be. Bad data is bad data. If you put rubbish in, you get rubbish out. No amount of AI is going to change that. Perhaps in the future it may, but right now it won’t.
When evaluating solutions to buy, it’s important to understand what data it will need access to. I have been privy to a great many demos and sales teams who are very experienced in showcasing the fantastic tricks that AI can do. Wondrous things. But then you scratch the surface and it becomes evident the demonstration was prepared and set up using specific data – data that either we don’t have, or we don’t want to make available. It’s business critical to have a grasp on what every solution needs access to and how it pertains to your specific data frameworks and use cases.
Another big challenge is that around copyrights. That’s the biggest concern for me. We’re deliberating here over open source versus buying, but many of the solutions that are on the market are built upon open-source technology. They’ve just done what Paul has, and then they’re selling it on – they're essentially shop-windowing ChatGPT or other solutions like that. Proprietary technology is not abundantly available and it’s incredibly expensive – look at the amount that OpenAI and others in the space are spending. Just how open source they are, we don’t know. And then you have open source tools that don’t disclose the training data or the methodologies that underpin their guardrails. That secrecy contradicts the ethos of open source.
While we endeavour to maintain impeccable data governance, there will be data questions marks over this technology for a long time.
SH: Moving on now to the human side of AI. This technology undoubtedly harbours tremendous potential to enable employee productivity growth. How are you both addressing the talent management piece of this equation?
DA: It’s a battle for hearts and minds.
Our colleagues are constantly coming across news articles suggesting AI will soon replace jobs – and naturally they’re apprehensive about the technology and they have concerns about redundancy. That being so, discussing the adoption of AI becomes a delicate matter – we certainly don’t want to demoralise or instill worry in our employees through implementation. We really look to espouse the benefits and how it can support people in their roles.
We focus on keeping the human-in-the-loop. It’s our people that make NRLA the organisation it is, and we’re trying to find ways to augment and assist them in not just one, but multiple processes through AI adoption – not replace them. And we hear it from our members – we're in the business of giving advice, and they want to hear that advice from a person.
I share Paul’s viewpoint that large language models are not yet ready to effectively engage with customers. They’re not there. You just can’t make any guarantees. Our people – and the process of empowering our people – is our priority.
PC: We've been on a similar journey. In the initial stages of AI maturation, there was a widespread perception that this technology would lead to job displacement. Some of the use cases we first selected may not have been the most effective in terms of demonstrating the potential of AI.
Once we shifted the narrative to highlight that AI is an assistant – a tool designed to enhance performance – the mood changed. We’re all knowledge workers. We’re all living in fear that AI could supplant our roles. But when you reframe it as an instrument to augment productivity, it starts to make more sense. The worst approach is to simply hide away from it and pretend an AI revolution isn’t happening – because it most definitely is. Early education around the correct mindset has been our focus.
SH: You are both set to speak at the CCW UK Executive Exchange in March, which promises to unpack the myriad ways in which brands can future-proof their CX strategies. Dan, the core subjects you’ll be addressing in your presentation are how to evaluate incumbent AI partners and how to build a business case for switching to a new vendor. As a prelude to that session, can you share some best practices around how to pitch the importance of CX-focused investment to the boardroom?
DA: My presentation will focus on the end-to-end journey, and I characterise it as a progression from simplicity to complexity and then back to simplicity. That’s the process we abide by.
We begin by identifying the problem we’re trying to solve. If you don’t understand that fundamental aspect of your transformation, you’ll likely make some missteps. What are your guiding principles? What are you hoping to achieve? The responses to these questions should be clearly and concisely documented. If you can’t explain what you’re doing in 30 seconds, you’re doing it wrong. You need to distill everything down – and that’s a process that can take time. It’s a real challenge.
Then get complicated. In a recent vendor transition, I created a list of 180 “must and shoulds” – items detailing what the technology has to do. You get under the metaphorical bonnet and understand what it must accomplish. Over a period, the realisation will dawn that you’ve overly complicated, and that’s when you make it simple again. If you’re trying to sell your board or other stakeholders an idea that’s convoluted, intricate, nuanced – a concept that isn’t immediately digestible – then that is a recipe for failure.
It becomes a reductive process, particularly when you’re pitching a relationship with a pre-selected partner or you’re pitching to go out to RFP. Boil it down: What are the key benefits for the organisation? And that should almost never come back to money. Returns are a significant element, of course – and it may even constitute 90% of the decision-making process: Naturally, it has to wash its face. But place the emphasis on how this proposition enhances the organisation, how it offers a competitive advantage, or – in our case – how it makes processes better for members.
Really break that down, Make it simple. No long drawn-out presentations. Communicate the rationale in two or three minutes and engage your audience in the journey.
SH: Paul, you’ll be running a think tank session focused on exploring how to create an ethical risk framework when leveraging open-source AI. To set the stage for that, can you describe the work you’ve done in this area? How did you ensure the AI models you leveraged were free of bias, devoid of hallucinations, and fully compliant with regulations?
PC: I’m going to dive into a specific use case related to the contact centre. I managed a large contact centre in which we had a quality control team responsible for assessing a percentage of calls to monitor handling – looking at professionalism, efficiency, etc. That’s standard for most contact centres, but we wanted to get granular. We hypothesised that implementing an AI model would allow us to listen to calls quicker and therefore provide our agents with more targeted and timely insights.
However, as we started getting deeper into the concept, we encountered some significant ethical questions. For example, if we tasked the AI with identifying the top five conversations from the previous day, it might repeatedly highlight the same top performers. The question arising, then, is how far do you go with those performance conversations? You’re effectively transferring the performance of your contact centre over to AI.
So, back to the think tank: I’ll be putting that use case out there and exploring the ethical forks in the road.
SH: Last question for you both to round us off. Reflecting on everything you’ve done and achieved with AI technology to date, what are some standout lessons from your experience that could guide others in similar roles?
DA: Talk to everyone. It's such a busy space. There are so many people running so many different initiatives. AI has been the hot topic in the customer experience industry for a couple of years now. Don't get overwhelmed by it. Solicit advice. Read. Immerse yourself in that world to form your independent conclusions and craft your own opinions around how to proceed. And approach the information shared with a critical mindset – many individuals and parties have vested interests. Be wary and mindful of the motive behind the conversations you’re having – oftentimes you’re being sold to but, of course, that doesn’t necessarily diminish the value of the insights.
Just get stuck in. Although it can feel overwhelming, and the associated risks may look like a real challenge, the best way to go is to engage with a multitude of people and take those first steps.
PC: I echo those points.
What we need to do with this technology is get involved. It’s essential that we, as an industry, understand its capabilities and understand what it’s about beyond mere marketing rhetoric. There’s so much great content out there – read up and arm yourself with some of the key concepts and dive into the detail. There’s a lot of opportunity.
Diverse perspectives and increased participation will only help us take on the associated risks and benefits of AI. And by fostering broader involvement, the necessary controls and regulations will naturally fall into place.